Applied Longitudinal Data Analysis by Judith D. Singer and John B. Willett – Summary and Review
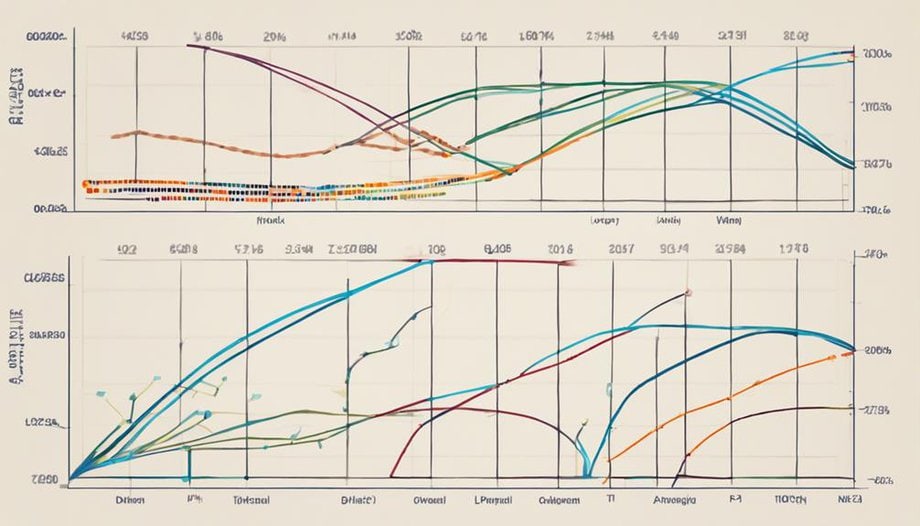
'Applied Longitudinal Data Analysis' by Judith D. Singer and John B. Willett is a comprehensive guide for researchers looking to understand student progress over time through longitudinal data analysis.
This book offers clear explanations of key concepts and practical applications across different fields, making it a valuable resource.
Singer and Willett's work has had a profound impact on longitudinal data analysis, despite some existing controversies and critiques.
Key Takeaways
- Offers valuable insights into analyzing data over time.
- Addresses challenges in interpreting complex longitudinal data.
- Influences research practices and methodological advancements.
- Shapes understanding of longitudinal data complexities for future research.
Overview of the Book
In exploring the essence of 'Applied Longitudinal Data Analysis,' one encounters a detailed guide that navigates the intricacies of longitudinal data with clarity and depth. The book provides a thorough overview of various data analysis techniques tailored for longitudinal research insights. Through a meticulous examination of longitudinal data, readers are equipped with the knowledge and tools necessary to analyze trends, patterns, and relationships over time.
By delving into the complexities of longitudinal research, the authors offer valuable insights into how to effectively capture the dynamic nature of data. From understanding the nuances of growth models to deciphering the intricacies of repeated measures analysis, the book serves as a beacon of knowledge for researchers seeking to unravel the mysteries hidden within longitudinal data.
With a keen focus on practical applications and theoretical foundations, 'Applied Longitudinal Data Analysis' emerges as an indispensable resource for anyone looking to harness the power of longitudinal data in their research endeavors.
Authors' Background and Expertise
The authors' background and expertise serve as the bedrock of their credibility in the field of longitudinal data analysis. Their qualifications, research experience, and academic achievements form a solid foundation upon which the book is built.
Through their shared expertise, readers are guided by seasoned professionals who bring a wealth of knowledge to their work.
Authors' Qualifications Highlighted
Emanating a wealth of academic and practical experience, the authors of 'Applied Longitudinal Data Analysis' exhibit a profound understanding of longitudinal data analysis methodologies.
- Distinguished Career: Both Singer and Willett are esteemed professors at Harvard University, renowned for their contributions to the field of statistics.
- Extensive Publication Record: With numerous publications in prestigious journals, their expertise is widely recognized in academia.
- Consultation Services: Through their consulting work, they've applied their knowledge to real-world research problems, enhancing their practical understanding of data analysis techniques.
Singer and Willett's qualifications underscore their authority in the domain of longitudinal data analysis, making their book a valuable resource for both researchers and practitioners seeking to explore further into this complex subject.
With a rich history of academic accomplishments and practical applications, Singer and Willett's research experience provides a robust foundation for their authoritative insights in longitudinal data analysis. Their combined expertise offers a unique blend of theoretical knowledge and hands-on involvement in real-world research projects. Here is a glimpse into their personal insights and approach to data interpretation:
Personal Insights | Data Interpretation |
---|---|
Extensive research in longitudinal methods | Advanced statistical techniques |
Focus on educational and psychological data | Complex models for data analysis |
Practical applications in various industries | Longitudinal data visualization |
Emphasis on interdisciplinary collaborations | Strategies for handling missing data |
Ongoing contributions to research methodology | Interpretation of growth curve models |
Academic Achievements Discussed
Amidst the landscape of scholarly accomplishments, the authors' background and expertise in longitudinal data analysis shine brightly, reflecting a profound dedication to advancing research methodologies. Judith D. Singer and John B. Willett have made significant contributions to the field, particularly in understanding academic success and educational outcomes through longitudinal data analysis.
Their expertise is evident in various aspects:
- Pioneering research in educational trajectories
- Developing innovative statistical methods for analyzing longitudinal data
- Influencing policy decisions by providing robust evidence on factors influencing academic achievement
Through their academic achievements, Singer and Willett haven't only deepened our understanding of educational outcomes but have also paved the way for future research in the field.
Key Concepts Explored
Through an intricate lens, the authors of 'Applied Longitudinal Data Analysis' dissect core statistical methods, guiding readers through the complex terrain of longitudinal data analysis.
They illuminate the practical applications of these methods, emphasizing their relevance in real-world scenarios.
The exploration of these key concepts serves as a beacon for those traversing the seas of longitudinal data analysis.
Core Statistical Methods
Delving into the core statistical methods of longitudinal data analysis reveals a sophisticated framework for uncovering dynamic trends and relationships over time.
- Multilevel Modeling: Allows for the analysis of nested data structures, capturing variability at different levels.
- Generalized Estimating Equations (GEE): Useful for analyzing data with correlation structures, providing population-average interpretations.
- Survival Analysis: Examines time-to-event data, essential for studying durations until a specific event occurs.
These statistical methods offer a powerful toolkit for researchers seeking to understand the complexities of longitudinal data. By applying these techniques, one can extract valuable insights and explore the dynamic nature of relationships embedded within the data, paving the way for enhanced decision-making and deeper understanding.
Longitudinal Data Analysis
Exploring the domain of Longitudinal Data Analysis uncovers a rich tapestry of key concepts essential for unraveling the dynamic trajectories within datasets. Data interpretation and statistical modeling are pivotal in extracting meaningful insights from longitudinal data. By delving into the patterns of change over time, researchers can grasp the underlying processes shaping the phenomena under study. Below is a table illustrating some fundamental aspects of Longitudinal Data Analysis:
Key Concepts | Description |
---|---|
Trajectory Analysis | Examining individual paths of change over time |
Growth Modeling | Capturing overall trends and patterns of growth |
Time-Varying Covariates | Understanding how predictors change over time |
Latent Growth Modeling | Uncovering underlying constructs driving change |
Understanding these concepts is vital for conducting rigorous longitudinal analyses and drawing robust conclusions from complex datasets.
Practical Applications
Revealing the practical applications of key concepts in longitudinal data analysis illuminates the transformative potential of harnessing dynamic data trajectories for informed decision-making and predictive modeling.
- Real-world examples: Demonstrating how longitudinal data analysis was used to predict customer churn rates in a telecom company.
- Case studies: Showcasing how longitudinal data analysis helped track the progress of students over time to identify factors influencing academic performance.
- Application in healthcare: Highlighting the use of longitudinal data analysis to understand the effectiveness of different treatments for chronic diseases.
These instances underscore the invaluable insights that can be gleaned from longitudinal data analysis, making it a powerful tool for understanding trends, making predictions, and guiding strategic actions in various fields.
Practical Applications in Research
Researchers often find practical applications in their studies that offer valuable insights into real-world phenomena. In the domain of practical research and data analysis, the utilization of longitudinal data provides a unique opportunity to uncover trends, patterns, and relationships over time. By examining data points collected at multiple intervals, researchers can gain a deeper understanding of how variables evolve and interact with each other. This approach enables them to track changes, predict future outcomes, and make informed decisions based on empirical evidence.
Practical applications in research extend beyond theoretical frameworks, allowing scholars to address complex issues with a more nuanced perspective. Through longitudinal data analysis, researchers can explore causal relationships, test hypotheses, and validate existing theories in a dynamic context. This methodological approach not only enhances the credibility of research findings but also provides actionable insights for policymakers, practitioners, and other stakeholders. By embracing longitudinal data analysis in their studies, researchers can bridge the gap between theory and practice, contributing meaningfully to the advancement of knowledge in various fields.
Impact on Longitudinal Data Analysis
The profound impact of longitudinal data analysis lies in its ability to reveal intricate patterns and dynamics unfolding over time. Longitudinal studies offer a unique perspective that allows researchers to explore deep into the complexities of data and extract meaningful insights that can shape our understanding of various phenomena.
Here are some key points to take into account:
- Methodological Challenges: Conducting longitudinal data analysis poses several methodological challenges, such as handling missing data, dealing with attrition, and selecting appropriate models for complex longitudinal structures.
- Data Interpretation: Longitudinal data analysis enables researchers to interpret data in a temporal context, highlighting how variables evolve over time and identifying causal relationships that may not be apparent in cross-sectional studies.
- Trends: By analyzing data longitudinally, researchers can uncover trends and patterns that may not be observable in static datasets, providing a more thorough view of how variables change and interact over time.
Addressing these challenges and harnessing the power of longitudinal data analysis can lead to more robust research findings and a deeper understanding of the dynamics underlying various phenomena.
Critique and Controversies
Longitudinal data analysis, despite its significant contributions, hasn't been immune to criticisms and controversies within the research community. These controversies often revolve around the interpretation of results and methodological debates. One of the central issues in longitudinal data analysis is the challenge of making sense of complex data patterns over time. Researchers may have differing views on how to best analyze and interpret these patterns, leading to controversial interpretations. Methodological debates also play a significant role in fueling these controversies, as researchers may disagree on the most appropriate techniques to use when analyzing longitudinal data.
Critiques of longitudinal data analysis can serve as a catalyst for growth and improvement in the field. By engaging in thoughtful discussions and debates surrounding controversial interpretations and methodological debates, researchers can refine their approaches and enhance the quality of their analyses. While controversies may persist, they ultimately contribute to the evolution and advancement of longitudinal data analysis as a valuable research tool.
Influence on the Field
With its enduring influence on the field, longitudinal data analysis has shaped the trajectory of research practices in profound ways. This impact extends beyond the immediate applications of the methodology and reaches into broader trends in research and academia.
- Methodological Advancements: Longitudinal data analysis has spurred methodological advancements by encouraging researchers to develop more sophisticated techniques for analyzing complex data structures over time. This has led to a deeper understanding of the dynamic nature of various phenomena under study.
- Interdisciplinary Collaborations: The approach of longitudinal data analysis has fostered interdisciplinary collaborations, bringing together experts from diverse fields to tackle complex research questions. This cross-pollination of ideas has enriched the research landscape and led to the emergence of novel perspectives and methodologies.
- Innovative Approaches: Researchers leveraging longitudinal data analysis have been at the forefront of developing innovative approaches to studying phenomena longitudinally. This has opened new avenues for exploration and paved the way for future directions in research that prioritize longitudinal data collection and analysis.
Conclusion and Recommendations
Having navigated through the domain of applied longitudinal data analysis, one can distill essential conclusions and offer insightful recommendations for future endeavors in this domain. To summarize, the key takeaways from Singer and Willett's work emphasize the importance of understanding the complexities of longitudinal data and the significance of analyzing data over time. Researchers should prioritize designing studies that capture longitudinal information accurately and utilize appropriate statistical methods to analyze this data effectively. Additionally, the authors stress the value of considering both within-individual changes and between-individual differences in longitudinal analysis to gain a thorough understanding of the phenomena under study.
Moving forward, it's recommended that researchers continue to explore innovative techniques for handling longitudinal data, including advanced modeling approaches that can account for the complexity of longitudinal datasets. Additionally, fostering collaborations between experts in different fields, such as statistics, psychology, and sociology, can lead to interdisciplinary advancements in longitudinal data analysis. By embracing these recommendations, researchers can enhance the quality and depth of insights derived from longitudinal studies, contributing to the advancement of knowledge in various domains.
Frequently Asked Questions
What Are Some Common Challenges Researchers Face When Working With Longitudinal Data Analysis and How Does This Book Address Them?
Researchers face challenges in longitudinal data analysis such as data management, model selection, and addressing missing data. This book offers solutions through thorough guidance on handling complex data structures, selecting appropriate models, and addressing common analytical hurdles.
Can You Provide Examples of Real-Life Research Studies Where the Concepts Discussed in the Book Were Successfully Applied?
Real-life case studies showcase the book's methodological applications. Researchers have successfully applied concepts from the book in diverse studies, highlighting its practical utility. The real-world implementation of these techniques underscores their relevance and effectiveness in research.
How Does This Book Compare to Other Textbooks on Longitudinal Data Analysis in Terms of Depth and Clarity of Explanation?
In comparing textbooks on longitudinal data analysis, depth and clarity are paramount. This book excels by offering a thorough blend of intricate details with clear explanations. It outshines competitors by striking a harmonious balance between complexity and understandability.
Are There Any Limitations to the Practical Applications of the Methods Discussed in the Book, and if So, How Can Researchers Overcome Them?
In research studies, limitations may arise in the practical applications of methods. Overcoming challenges involves adapting techniques to suit specific data sets, considering assumptions critically, and utilizing complementary approaches for robust analyses.
How Have Advancements in Technology and Data Collection Methods Influenced the Field of Longitudinal Data Analysis Since the Publication of This Book?
Advancements in technology and data collection methods revolutionized longitudinal data analysis post-publication. Researchers face challenges in real-life studies due to limitations in practical applications. Overcoming these obstacles involves adapting to new tools, refining comparisons, and enhancing data interpretation.