Applied Regression Analysis and Generalized Linear Models by John Fox – Summary and Review
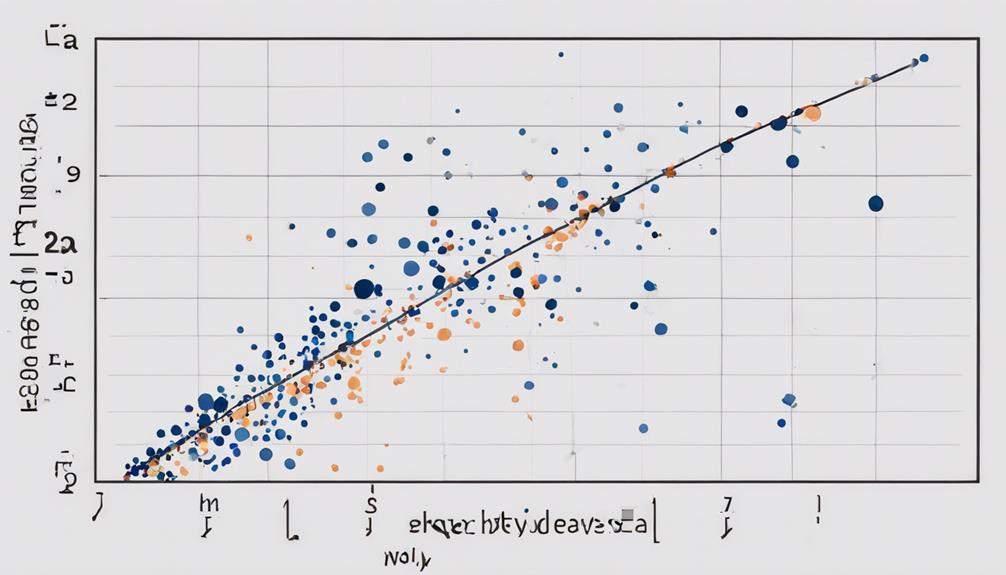
These classic texts offer a comprehensive approach to regression techniques, covering both theoretical foundations and practical applications. Fox's clear explanations and detailed examples make complex statistical concepts accessible to readers at all levels of expertise. His emphasis on interpretation and visualization of results enhances the reader's ability to draw meaningful conclusions from regression analyses.
Overall, John Fox's books serve as essential resources for researchers, students, and practitioners seeking to master regression analysis and apply it effectively in their work.
Key Takeaways
- Comprehensive coverage of regression analysis and GLMs with practical examples
- Emphasis on model interpretation, assumptions testing, and residual analysis
- Versatile toolkit for diverse applications across industries
- Enhances decision-making with data-driven insights and rigorous research outcomes
Book Overview
Upon delving into the book, readers will find a thorough overview of regression analysis and generalized linear models. The book is meticulously organized, presenting complex statistical methods in a clear and accessible manner. Each chapter flows seamlessly into the next, building upon previously introduced concepts to provide a detailed understanding of regression analysis.
The book's organization is designed to guide readers through a logical progression of topics, starting from the fundamental principles of regression analysis and gradually delving into more advanced techniques. Through a structured approach, readers are able to grasp the intricacies of generalized linear models and their applications in real-world scenarios.
The book not only explains the theoretical foundations of regression analysis but also provides practical examples and case studies to illustrate how these statistical methods can be implemented in research and data analysis. By combining theoretical knowledge with hands-on experience, the book equips readers with the tools necessary to apply regression analysis effectively in their own work.
Regression Analysis Concepts
Exploring the fundamental concepts of regression analysis reveals a foundational understanding of statistical modeling techniques. In this domain, managing multicollinearity is vital to prevent misleading results. Model interpretation plays a pivotal role in extracting meaningful insights from the data and translating them into actionable decisions. Assumptions testing is a necessary step to ascertain the validity of the regression model, as violating these assumptions can lead to inaccurate conclusions. Additionally, conducting thorough residual analysis aids in evaluating the goodness-of-fit and identifying any patterns or outliers that could impact the model's performance.
Effective regression analysis involves not only fitting a model to the data but also scrutinizing its robustness and reliability. By delving into the intricacies of multicollinearity management, model interpretation, assumptions testing, and residual analysis, practitioners can refine their regression models and enhance the accuracy of their predictions. This deeper understanding empowers analysts to make informed choices and extract valuable insights from their data with confidence.
Generalized Linear Models Introduction
Entering the domain of Generalized Linear Models introduces analysts to a sophisticated framework for statistical modeling beyond traditional regression analysis.
Generalized Linear Models (GLMs) expand the scope of regression by accommodating a more extensive range of response variables and error distributions, making them powerful tools for various data analysis tasks.
Unlike ordinary linear regression, GLMs can handle non-normally distributed data and model relationships between variables using different types of probability distributions. This flexibility allows analysts to work with count data, binary outcomes, and other types of responses that may not fit the assumptions of classical linear regression.
By incorporating concepts such as link functions and generalized estimating equations, GLMs provide a robust foundation for exploring complex relationships within datasets.
Embracing Generalized Linear Models opens up new avenues for statistical modeling, empowering analysts to peer deeper into the intricacies of their data and derive meaningful insights that traditional regression techniques may overlook.
Applications in Research
Venturing into the domain of Generalized Linear Models enables researchers to uncover diverse and innovative applications in their research endeavors. These models offer a versatile toolkit that can be applied across various fields, including education and research methodology.
- Applications in Education: Generalized Linear Models can be used to analyze educational data such as student performance scores, the effectiveness of teaching methods, or factors influencing dropout rates. Researchers can employ these models to gain insights into complex educational phenomena and make data-driven decisions to improve learning outcomes.
- Research Methodology: In the domain of research methodology, Generalized Linear Models provide a robust framework for analyzing data with different distributions and relationships. Researchers can utilize these models to investigate hypotheses, identify patterns, and draw meaningful conclusions from their research findings. By incorporating these models into their methodological approaches, researchers can enhance the rigor and validity of their studies, leading to more credible and impactful research outcomes.
Strengths of the Book
Revealing the book's strengths shows a thorough and insightful guide to Regression Analysis and Generalized Linear Models. The strengths of 'Applied Regression Analysis and Generalized Linear Models' by John Fox lie in its extensive examples and detailed explanations. This book excels in providing readers with a deep understanding of regression analysis and generalized linear models through a combination of theory and practical application.
Below is a table highlighting some of the specific strengths of the book:
Strengths | Description |
---|---|
Extensive Examples | The book offers a wide range of examples from various fields, aiding in the practical application of concepts. |
Detailed Explanations | Fox provides thorough explanations that help readers grasp complex topics with clarity and depth. |
Practical Guidance | The book not only explains the theories but also offers practical guidance on implementing these models in real-world scenarios. |
These strengths make 'Applied Regression Analysis and Generalized Linear Models' a valuable resource for both beginners and experienced practitioners in the field of regression analysis.
Limitations and Criticisms
The book's exploration of limitations and criticisms sheds light on areas where 'Applied Regression Analysis and Generalized Linear Models' may fall short. While the text offers valuable insights and techniques, it's essential to acknowledge some critiques and methodological concerns to provide a balanced view for readers.
- Critiques: Some readers find the book's explanations complex, potentially hindering understanding for beginners.
- Alternatives: It may be beneficial to explore additional resources or supplementary texts to gain a more thorough understanding of the subject matter.
- Statistical Assumptions: The book's reliance on certain statistical assumptions could be a limitation in practical applications, where real-world data may not always adhere to these assumptions.
Taking these factors into account can help readers navigate the content more effectively and make informed decisions when applying regression analysis and generalized linear models in their work.
Practical Use Cases
Exploring the practical use cases of regression analysis and generalized linear models reveals their real-world applications across various industries. Understanding their relevance in different sectors can illuminate the role they play in problem-solving scenarios, offering valuable insights and predictive capabilities.
Real-World Applications
In various industries, practitioners leverage regression analysis and generalized linear models to uncover insights and make informed decisions based on data analysis. These statistical tools find practical applications in a variety of real-world examples and case studies:
- Forecasting sales trends in retail businesses based on historical data.
- Analyzing the impact of marketing campaigns on customer engagement and conversion rates.
- Predicting patient outcomes in healthcare settings to optimize treatment plans and resource allocation.
These hands-on experiences showcase the versatility of regression analysis and generalized linear models in addressing complex problems and guiding strategic decision-making processes across different sectors.
Industry Relevance
Practitioners across diverse industries seamlessly integrate regression analysis and generalized linear models into their decision-making processes, showcasing the practical relevance and impact of these statistical tools in real-world settings.
These methods have found wide industry applications, from finance to healthcare, marketing to engineering. Case studies abound where regression analysis has been instrumental in predicting market trends, optimizing manufacturing processes, or understanding patient outcomes.
In finance, generalized linear models assist in credit scoring and risk analysis, while in marketing, they aid in customer segmentation and predicting campaign success. The versatility of these tools makes them indispensable for businesses seeking data-driven insights to drive strategic decision-making and stay ahead in competitive market landscapes.
Problem-Solving Scenarios
Amidst the complexity of modern business challenges, regression analysis and generalized linear models emerge as guiding beacons, illuminating paths to effective problem-solving solutions. When considering practical examples of problem-solving scenarios, these statistical techniques prove invaluable:
- Marketing Campaign Optimization: Utilizing regression analysis to determine the impact of different marketing strategies on sales.
- Risk Assessment in Finance: Applying generalized linear models to predict the likelihood of default on loans based on various financial indicators.
- Healthcare Resource Allocation: Using regression analysis to optimize the allocation of medical resources based on patient demographics and needs.
These problem-solving techniques showcase the versatility and power of regression analysis and generalized linear models in addressing a wide array of real-world challenges.
Final Thoughts
As one reflects on the key takeaways, the essence of the regression analysis and generalized linear models comes into focus.
The overall evaluation of the book underlines the importance of a deep understanding of statistical methods in today's data-driven world.
These insights have direct implications for improving one's practice and decision-making processes.
Key Takeaways Highlighted
What're the key insights that emerge when reflecting on Regression Analysis and Generalized Linear Models? When diving into the complexities of statistical techniques and data analysis, certain takeaways come to light:
- Importance of Model Assumptions: Acknowledging and testing the assumptions underlying regression models is essential for accurate results.
- Interpretation of Coefficients: Understanding the coefficients' significance and direction can provide valuable insights into the relationships within the data.
- Model Selection Process: The process of selecting the most appropriate model involves balancing simplicity with accuracy to make sure the best fit for the data at hand.
Overall Book Evaluation
Reflecting on the insights gained from exploring Regression Analysis and Generalized Linear Models, the overall evaluation of the book brings together a thorough understanding of statistical techniques and their practical applications. Through critical analysis, the book not only explores into the theoretical aspects of regression but also provides a bridge to apply these concepts in real-world scenarios.
The evaluative insights offered are invaluable for both beginners and experienced professionals in the field of statistics. Readers will appreciate the clear explanations and practical examples that aid in grasping complex concepts with ease. John Fox's work stands out for its ability to simplify intricate statistical methods, making them accessible and relevant.
This book serves as a detailed resource for anyone looking to master regression analysis and generalized linear models.
Implications for Practice
In considering the implications for practice, practitioners will find that the insights from Regression Analysis and Generalized Linear Models offer a practical roadmap for applying statistical techniques effectively in real-world settings. These models have significant implications for practical applications and data analysis, providing a structured approach to interpreting and utilizing data.
By understanding statistical modeling and predictive analytics, professionals can make informed decisions and predictions based on data patterns. This knowledge empowers practitioners to extract valuable insights, identify trends, and forecast outcomes with confidence.
Embracing these methodologies can enhance decision-making processes and lead to more informed strategies in various fields where data analysis plays a critical role.
Frequently Asked Questions
How Does the Book Address Potential Biases in Regression Analysis and Generalized Linear Models?
In addressing biases, the book tackles multicollinearity challenges by providing insightful strategies. It emphasizes the importance of identifying and mitigating potential biases to enhance the accuracy and reliability of regression analysis and generalized linear models.
Does the Book Provide Any Guidance on Dealing With Multicollinearity in Regression Analysis?
In dealing with multicollinearity, the book offers guidance through diagnostic checks to assess the issue's impact. By examining correlations among predictors, users can identify and address potential challenges in regression analysis effectively.
Are There Any Discussions on the Assumptions Underlying Generalized Linear Models and Their Implications for Analysis?
Delving into the assumptions of generalized linear models sheds light on their implications for analysis. Understanding these model biases is essential for accurate interpretations. Awareness of these aspects enriches the analytical process and enhances the validity of results.
How Does the Book Handle Missing Data and Its Impact on Regression Analysis and Generalized Linear Models?
In handling missing data, the book explores various imputation strategies to address this common challenge. It delves into the impact of missing data on model performance and emphasizes the importance of conducting sensitivity analysis for robust results.
Does the Book Offer Any Insights Into the Practical Challenges of Implementing Regression Analysis and Generalized Linear Models in Real-World Research Settings?
In handling practical implementation challenges, the book explores real-world research insights, offering valuable perspectives. It sheds light on the complexities of applying regression analysis and generalized linear models effectively in diverse research settings.