Multilevel and Longitudinal Modeling Using Stata by Sophia Rabe-Hesketh – Summary and Review
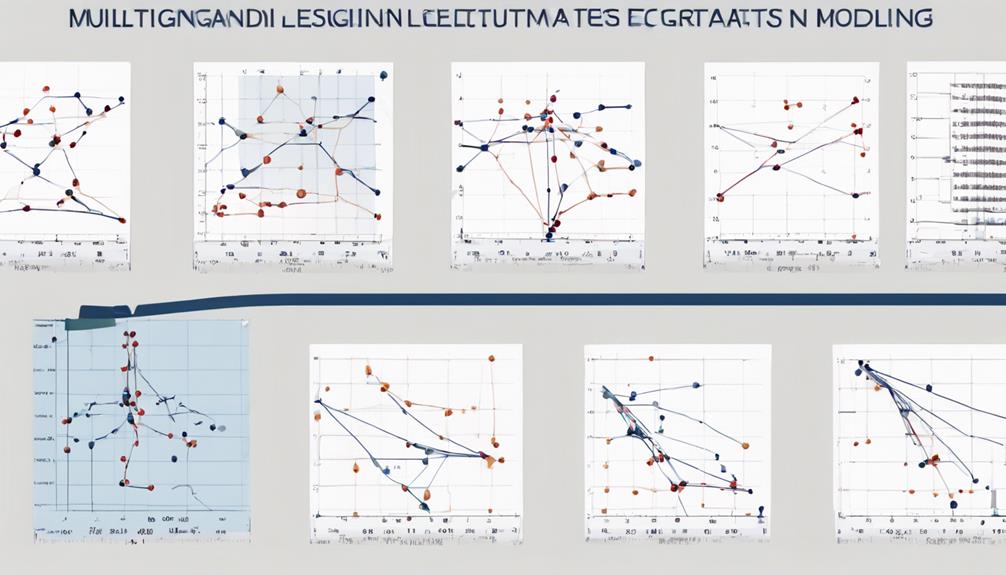
Sophia Rabe-Hesketh's book on multilevel and longitudinal modeling offers a sophisticated framework for analyzing complex data structures.
It provides advanced statistical techniques and practical applications, making it a must-have resource for researchers and analysts.
Those looking to elevate their data analysis skills and gain invaluable insights will find this book to be a valuable asset.
Key Takeaways
- Multilevel modeling in Stata captures hierarchical data structures effectively.
- Longitudinal modeling in Stata studies temporal changes within subjects over time.
- Combining both approaches provides insights into individual and group factors interactions.
- HLM, SEM, and Growth Curve Modeling in Stata offer comprehensive analysis of hierarchical and temporal data.
Overview of Multilevel and Longitudinal Modeling
In the domain of advanced statistical analysis, multilevel and longitudinal modeling stand as pillars of insight and understanding, illuminating the intricate relationships within data over time. Multilevel modeling, often referred to as hierarchical linear modeling, allows researchers to analyze data that has a hierarchical structure, such as students nested within schools or patients within hospitals. On the other hand, longitudinal modeling focuses on studying changes within the same subjects over a period. By combining these two approaches, researchers can capture the complexity of data that evolves both hierarchically and temporally.
The benefits of utilizing multilevel and longitudinal modeling are profound. They offer a nuanced perspective on how individual and group-level factors interact and influence outcomes over time. This framework not only provides a thorough understanding of the data but also enables the identification of trends and patterns that might be overlooked in traditional analyses. The implementation of these models requires a solid grasp of statistical theory and methodology, ensuring that researchers can navigate the intricacies of complex data structures with confidence and precision.
Key Concepts and Methodologies Explored
Upon delving into the domain of multilevel and longitudinal modeling, one encounters a rich tapestry of key concepts and methodologies that weave together to disclose profound insights into data dynamics. Advanced methodologies such as Hierarchical Linear Modeling (HLM), Structural Equation Modeling (SEM), and Growth Curve Modeling are pivotal in understanding the complexities of nested data structures and temporal trends within datasets. These techniques provide a robust framework for analyzing data where observations are not independent and evolve over time. Additionally, concepts like random effects, fixed effects, intra-class correlation, and autoregressive processes play an essential role in capturing the nuances of hierarchical data and longitudinal patterns. The table below summarizes some key concepts and methodologies explored in the domain of multilevel and longitudinal modeling:
Key Concepts | Methodologies |
---|---|
Random Effects | Hierarchical Linear Modeling (HLM) |
Fixed Effects | Structural Equation Modeling (SEM) |
Intra-class Correlation | Growth Curve Modeling |
Autoregressive Processes | Longitudinal Data Analysis |
Multilevel Structures | Time-varying Covariates |
Practical Applications in Data Analysis
Embarking on the world of data analysis, one uncovers a plethora of practical applications that illuminate the intricate relationships within datasets. When delving into this domain, individuals will find that:
- Data Visualization: Utilizing tools like scatter plots, histograms, and heat maps can bring data to life, allowing for easier pattern recognition and insightful storytelling.
- Model Interpretation: Understanding how to interpret the results of statistical models is crucial. This includes grasping coefficients, standard errors, and p-values to draw meaningful conclusions from the data.
- Predictive Analytics: Applying models to predict future trends or outcomes based on historical data can guide decision-making processes and enhance strategic planning efforts.
In the domain of data analysis, honing skills in data visualization, mastering model interpretation, and leveraging predictive analytics are instrumental in unraveling the mysteries hidden within datasets. By embracing these practical applications, one can navigate the complexities of data with confidence and clarity, paving the way for informed decision-making and impactful insights.
Advanced Statistical Techniques Covered
Within the domain of advanced statistical techniques, model complexity is a key facet that demands attention. These intricate models require a nuanced understanding to interpret and apply them effectively.
Through practical application examples, the complexity can be unraveled, shedding light on their utility in real-world data analysis scenarios.
Model Complexity Explained
Exploring the domain of advanced statistical techniques requires a keen understanding of model complexity and its intricate layers. When delving into this domain, individuals encounter a tapestry of sophistication that demands adept navigation. Here are three key points to illuminate the essence of model complexity:
- Model Interpretation: Revealing the true meaning behind the statistical models is akin to deciphering a cryptic code, where each coefficient and interaction holds significance.
- Data Visualization: Transforming raw data into insightful visuals is an art form that illuminates patterns and trends, aiding in unraveling the complexities embedded within the data.
- Balancing Act: Striking a harmonious equilibrium between model intricacy and interpretability is essential for crafting models that are both robust and comprehensible.
Practical Application Examples
Delving into the domain of advanced statistical techniques reveals a landscape where intricate models come to life through practical application examples. Real-world examples and hands-on exercises play a pivotal role in understanding the complexities of multilevel and longitudinal modeling. By immersing oneself in these practical scenarios, one can grasp the nuances of applying these sophisticated statistical techniques in various research settings. Through these exercises, individuals can witness firsthand how different factors interact within a model and how to interpret the results effectively. Below is a table exemplifying the importance of practical application examples in mastering advanced statistical techniques:
Benefits of Practical Application Examples | Significance |
---|---|
Enhanced Understanding | Clear Conceptualization |
Application in Research Settings | Real-world Relevance |
Improved Interpretation | Effective Analysis |
Traversing through intricate data structures demands a keen understanding of their interconnected components and relationships. When faced with complex data arrangements, individuals must navigate with precision and insight to derive meaningful conclusions. Here are three essential considerations for successfully maneuvering through intricate data structures:
- Data Visualization: Utilize visual representations to uncover patterns, trends, and anomalies within the data. Visualization tools can aid in identifying relationships that may not be apparent through traditional data analysis methods.
- Model Interpretation: Develop a deep comprehension of the models used to analyze the data. Understanding the underlying assumptions and mechanisms of a model is essential for accurate interpretation and inference.
- Relationship Mapping: Establish clear connections between different elements of the data structure. Mapping out relationships can elucidate how variables interact and influence outcomes, providing a holistic view of the dataset.
Insights for Researchers and Analysts
Exploring intricate data structures demands a meticulous understanding of their interconnected components and relationships, setting the stage for researchers and analysts to uncover profound insights. By employing advanced modeling strategies, researchers can extract valuable information that may remain hidden within complex datasets. Through the lens of multilevel and longitudinal modeling using Stata, analysts can investigate into the dynamics of data over time and across different levels, providing a detailed view of the underlying processes at play.
Effective data interpretation is key in this endeavor, as it allows researchers to translate raw information into meaningful findings. By carefully considering the nuances of the data and employing appropriate modeling techniques, analysts can reveal patterns, trends, and relationships that offer valuable insights for decision-making and further research. Embracing a holistic approach to data analysis, researchers can reveal the full potential of their datasets, paving the way for new discoveries and a deeper understanding of the phenomena under study.
Enhancing Data Analysis Skills
Enhancing one's skills in data analysis requires a diligent commitment to mastering statistical techniques and honing the ability to derive meaningful insights from complex datasets. To truly excel in data analysis, one must embrace the following principles:
- Data Visualization: Utilize graphs, charts, and interactive visuals to represent data in a compelling and easily understandable manner. Visualization not only aids in communicating findings but also in exploring the underlying patterns within the data.
- Statistical Inference: Develop a deep understanding of statistical methods to draw reliable conclusions from data. Statistical inference allows analysts to make predictions, test hypotheses, and quantify uncertainty, providing a solid foundation for decision-making.
- Continuous Learning: Stay updated with the latest trends and advancements in data analysis. Enrolling in courses, attending workshops, and engaging in practical projects can further refine one's analytical skills and broaden their expertise in handling diverse datasets.
Value of 'Multilevel and Longitudinal Modeling
Embracing multilevel and longitudinal modeling offers researchers a methodological advantage by allowing them to analyze data that exhibit complex hierarchical structures and temporal dependencies.
Through these advanced statistical techniques, researchers can uncover nuanced insights that traditional models might overlook, enabling a deeper understanding of the phenomena under investigation.
The practical applications of these modeling approaches are diverse, spanning fields such as social sciences, epidemiology, and education, highlighting their versatility and significance in modern research endeavors.
Methodological Advantages of Multilevel Modeling
The inherent value of multilevel and longitudinal modeling lies in its ability to uncover nuanced relationships within complex data structures, providing a deeper understanding of the phenomena under study.
- Hierarchical modeling: Allows for the examination of how individual characteristics interact within larger group contexts, offering insights into both individual and group-level effects.
- Nested data analysis: Permits the exploration of data organized in a hierarchical manner, acknowledging dependencies and interactions at different levels of the hierarchy.
- Enhanced model flexibility: Enables the incorporation of various sources of variability, capturing the complexity of real-world phenomena more accurately.
Longitudinal Data Complexities
Revealing the complexities inherent in longitudinal data highlights the invaluable role of multilevel and longitudinal modeling in capturing the dynamic nature of phenomena over time. Longitudinal analysis involves studying subjects over multiple time points, leading to rich datasets that require careful organization. Data organization in longitudinal studies is essential to maintain the integrity and coherence of the information gathered. It allows researchers to track changes within individuals or groups, providing insights into trends, patterns, and causal relationships. By employing multilevel modeling techniques, researchers can effectively navigate the intricacies of longitudinal data, uncovering hidden dynamics and offering a deeper understanding of how variables evolve over time.
Benefits of Longitudinal Analysis | Challenges in Longitudinal Data | Importance of Data Organization |
---|---|---|
– Captures changes over time | – Missing data points | – Ensures data integrity |
– Examines trends and patterns | – Attrition of participants | – Facilitates meaningful analysis |
– Reveals causal relationships | – Time-varying covariates | – Enables tracking of changes |
Practical Applications in Research
In revealing the full potential of research endeavors, the utilization of multilevel and longitudinal modeling emerges as a pivotal tool for discerning intricate patterns and relationships within dynamic datasets.
Practical Applications in Research:
- Uncovering Hidden Trends: Multilevel modeling allows researchers to identify trends that may not be apparent in traditional analyses, providing deeper insights into complex phenomena.
- Understanding Individual Variation: Longitudinal modeling enables the exploration of how individuals change over time, offering a nuanced understanding of growth trajectories and variations within populations.
- Predicting Future Outcomes: Through practical examples in real-world research, these modeling techniques can forecast future trends, aiding in decision-making processes and policy implementations.
Frequently Asked Questions
How Does Stata Compare to Other Statistical Software Programs When It Comes to Conducting Multilevel and Longitudinal Modeling?
When comparing statistical software programs for multilevel and longitudinal modeling, Stata stands out for its user-friendly interface and robust capabilities. Its efficiency and versatility make it a popular choice among researchers and analysts.
Are There Any Common Pitfalls or Challenges That Researchers May Encounter When Using Multilevel and Longitudinal Modeling in Stata?
Researchers often face common challenges when using multilevel and longitudinal modeling in Stata. Properly handling missing data, selecting appropriate models, and mastering data manipulation techniques are essential for accurate and insightful analysis.
Can Multilevel and Longitudinal Modeling in Stata Be Applied to Non-Traditional Data Sources, Such as Social Media Data or Geospatial Data?
Multilevel and longitudinal modeling in Stata can indeed be applied to non-traditional data sources like social media data for insightful social media analysis and geospatial modeling, enabling researchers to explore new dimensions of data intricacies.
Are There Any Specific Guidelines or Best Practices for Interpreting and Reporting Results From Multilevel and Longitudinal Models in Stata?
When interpreting results from multilevel and longitudinal models in Stata, researchers should adhere to established reporting guidelines. Clear and concise explanations of findings enhance the credibility and impact of the study, fostering transparent communication within the scientific community.
How Does the Use of Multilevel and Longitudinal Modeling in Stata Contribute to the Broader Field of Statistical Analysis and Research Methodology?
In the domain of statistical innovation, the utilization of multilevel and longitudinal modeling in Stata enriches research methodology, offering nuanced insights into complex data structures and dynamic relationships, fostering a deeper understanding of phenomena.