Principles of Biostatistics by Marcello Pagano and Kimberlee Gauvreau – Summary and Review
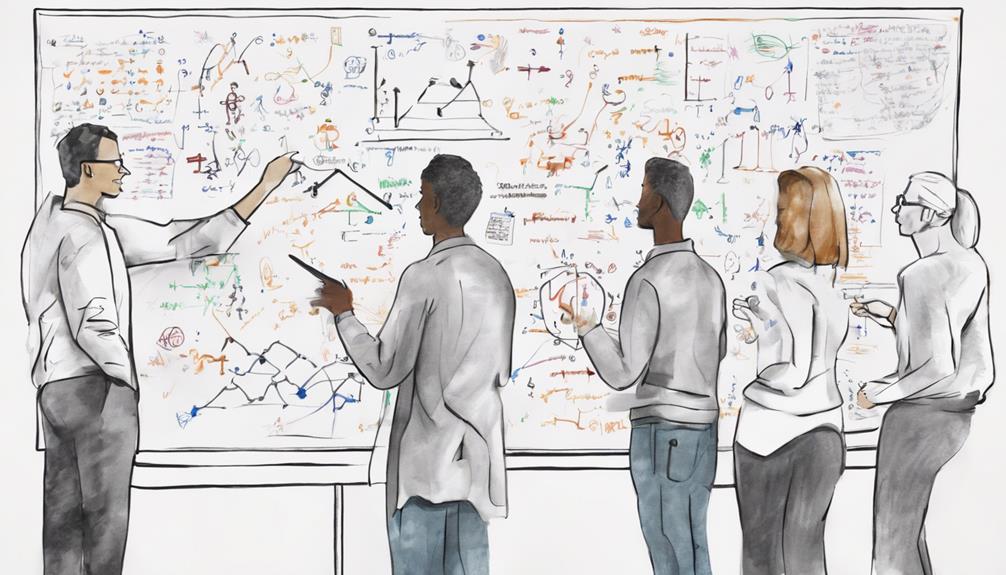
Marcello Pagano and Kimberlee Gauvreau's book on biostatistics emphasizes the critical role of sample size in study reliability, essential for public health and medical research.
Their work offers clear insights into complex statistical concepts, making it a valuable resource for data analysis in the field of biostatistics.
Key Takeaways
- Statistical inference aids in drawing conclusions from sample data for populations.
- Descriptive statistics effectively summarize complex dataset features for analysis.
- Biostatistics applications include clinical trials, epidemiological studies, and health outcomes research.
- Interactive learning methods and practical problem-solving enhance statistical comprehension and application in healthcare.
Author Background
Marcello Pagano and Kimberlee Gauvreau, the authors of 'Principles of Biostatistics,' bring a wealth of knowledge and experience to the field of study through their exceptional author collaboration. Pagano, a prominent biostatistician, is known for his groundbreaking work in statistical methods for clinical trials and epidemiology. His academic background includes a Ph.D. in Statistics from the University of London and a Master's degree in Mathematics from the University of Padua.
Gauvreau, on the other hand, complements Pagano's expertise with her strong foundation in biostatistics and epidemiology. She holds a Ph.D. in Biostatistics from Harvard University and has contributed to the development of statistical methods for public health research.
Together, Pagano and Gauvreau's academic backgrounds create a dynamic synergy that's reflected in the detailed and importantly insightful content of 'Principles of Biostatistics.' Their collaborative efforts have produced a masterpiece that not only educates but also empowers readers to navigate the complex world of biostatistics with confidence and freedom.
Overview of Content
With a thorough scope and meticulous attention to detail, 'Principles of Biostatistics' offers readers a profound exploration of key concepts and methodologies in the field. The book begins by laying a solid foundation in statistical reasoning, emphasizing the importance of study design and data collection. It then explores essential topics such as hypothesis testing, regression analysis, and survival analysis, providing a detailed overview of statistical methods commonly used in biostatistics.
To help readers grasp these intricate concepts, the authors skillfully integrate real-world examples and practical exercises throughout the text. This approach not only enhances understanding but also encourages critical thinking and application of statistical techniques in diverse research settings. Below is a table summarizing the key areas covered in 'Principles of Biostatistics':
Chapter | Key Concepts | Methodologies |
---|---|---|
Study Design | Randomization, Bias, Confounding | Experimental vs. Observational |
Hypothesis Testing | Null Hypothesis, p-values, Type I/II errors | t-tests, ANOVA, Chi-square tests |
Regression Analysis | Linear regression, Logistic regression | Model building, Interpretation |
Through this overview, readers gain a glimpse into the rich content awaiting them in 'Principles of Biostatistics', setting the stage for a fulfilling journey through the field of biostatistical analysis.
Key Statistical Concepts
In exploring the domain of biostatistics, understanding key statistical concepts serves as the cornerstone for unraveling the intricacies of data analysis and interpretation. These concepts form the bedrock upon which researchers build their understanding of the relationships within datasets and make informed decisions based on evidence.
Here are three essential elements to grasp in the domain of biostatistics:
- Statistical Inference and Hypothesis Testing: Statistical inference allows researchers to draw conclusions about a population from sample data, while hypothesis testing provides a framework for making decisions based on the likelihood of observing a particular outcome.
- Data Visualization: Visual representation of data is vital for gaining insights and communicating findings effectively. Through graphs, charts, and plots, complex datasets can be distilled into understandable patterns.
- Descriptive Statistics: Descriptive statistics summarize and describe the main features of a dataset, providing a snapshot of the data's characteristics without making inferences beyond the sample. This foundational knowledge is essential for further statistical analysis and interpretation.
Practical Applications
Practical applications of biostatistics illuminate the real-world impact of statistical analyses on healthcare decisions and public health policies. Data interpretation and statistical reasoning play pivotal roles in deriving meaningful insights from research findings and guiding evidence-based practices. By applying statistical methods to healthcare data, researchers and policymakers can make informed decisions that ultimately shape the well-being of individuals and communities.
Practical Application | Description | Importance |
---|---|---|
Clinical Trials | Evaluate treatment effectiveness in controlled settings | Critical for establishing medical interventions |
Epidemiological Studies | Investigate patterns of disease occurrence in populations | Inform public health strategies |
Health Outcomes Research | Assess the impact of healthcare services on patient outcomes | Enhance quality of care delivery |
These examples demonstrate how biostatistics transcends theoretical concepts to yield tangible benefits, driving advancements in healthcare and public health. Through rigorous data analysis and interpretation, statistical reasoning empowers stakeholders to make sound decisions that positively influence the lives of individuals and the prosperity of communities.
Pedagogical Approach
An effective pedagogical approach illuminates the path to mastering the intricate domain of biostatistics, guiding learners toward a profound understanding of statistical principles and their practical applications in healthcare and public health. When it comes to this challenging subject, interactive learning and hands-on activities play a pivotal role in enhancing comprehension and retention.
Here are three key elements that make the pedagogical approach in biostatistics impactful:
- Interactive Learning: Encouraging active participation through discussions, group activities, and simulations fosters engagement and deepens understanding.
- Hands-On Activities: Practical exercises, such as analyzing real-world healthcare data sets or conducting experiments, provide learners with valuable experience in applying statistical methods.
- Visual Aids and Technology: Utilizing visual aids like charts, graphs, and software tools not only makes complex concepts more accessible but also allows for a dynamic learning experience that resonates with modern learners.
Incorporating these components into the pedagogical approach can transform the study of biostatistics into an enriching and empowering journey towards statistical proficiency.
Illustrative Examples
In the domain of biostatistics, illustrative examples serve as beacons of practical problem-solving, shedding light on the real-world applications of statistical methods. Through these examples, complex statistical concepts are illuminated, empowering learners to grasp the essence of data analysis within the context of biology and medicine.
Real-World Applications
Wisdom gleaned from the principles of biostatistics finds its truest meaning when applied to real-world scenarios, illuminating the path to informed decision-making in healthcare and research. In the domain of medical research and public health, statistical methods play a pivotal role in shaping policies, guiding interventions, and improving outcomes. Here are three illustrative examples of how biostatistics is crucial in real-world applications:
- Clinical Trials: Biostatistics guarantees the rigorous design and analysis of clinical trials, determining the safety and efficacy of new treatments.
- Epidemiological Studies: Statistical techniques help uncover patterns of disease spread, identify risk factors, and evaluate preventive strategies in public health.
- Health Policy Analysis: Biostatisticians provide data-driven insights to policymakers, aiding in the development of effective healthcare policies and interventions.
Practical Problem-Solving
Harnessing the power of biostatistics in practical problem-solving illuminates the path to insightful solutions in healthcare and research endeavors. Employing problem-solving strategies and applying analysis techniques are pivotal in unraveling complex issues within these domains.
Case studies serve as beacons of practical application, showcasing how statistical methodologies can be wielded to address real-world challenges effectively. By delving into these examples, one can grasp the essence of utilizing biostatistics for informed decision-making.
The amalgamation of theoretical knowledge with hands-on problem-solving experiences cultivates a deeper understanding of the subject matter. Through the lens of biostatistics, individuals are empowered to navigate the intricacies of healthcare and research landscapes with precision and confidence, fostering a culture of innovation and progress.
Exercises and Problems
Exploring the exercises and problems in biostatistics provides a practical approach for mastering statistical concepts and applications. Engaging with these challenges not only sharpens one's analytical skills but also fosters a deeper understanding of data interpretation and statistical modeling.
Here are three essential aspects for delving into biostatistical exercises:
- Real-World Scenarios: The exercises often present real-life situations where statistical methods are applied to solve practical problems. This provides learners with a hands-on experience of how biostatistics is used in various research settings, enhancing their ability for applying these techniques in their own work.
- Critical Thinking: Tackling the problems requires critical thinking and logical reasoning for analyzing data, identifying patterns, and drawing meaningful conclusions. This process helps individuals develop a structured approach for problem-solving, which is invaluable in the field of biostatistics.
- Validation of Understanding: Successfully completing exercises validates one's comprehension of key statistical concepts and methodologies. It serves as a self-assessment tool for gauging proficiency and identifying areas for improvement, guiding learners towards mastery of biostatistics.
Impact on Biostatistics
Advancements in statistical methods have profoundly influenced the field of biostatistics, shaping the way researchers design studies and analyze data.
The implications extend beyond just numbers; they impact the very core of research design and decision-making processes.
This evolution signifies a shift towards more robust, precise, and insightful ways of understanding complex biological phenomena.
Statistical Methods Advancement
The evolution of statistical methods continues to shape the landscape of biostatistics, ushering in a new era of precision and insight. These advancements have brought about significant changes in the field:
- Increased Efficiency: New statistical methods allow for quicker analysis and interpretation of complex data sets, enabling researchers to draw conclusions faster.
- Enhanced Accuracy: Statistical modeling techniques have improved the accuracy of predictions and estimates, leading to more reliable results in studies involving health and medicine.
- Better Decision Making: Improved data interpretation methods facilitate better decision-making processes in healthcare by providing clearer insights into trends and outcomes, ultimately enhancing patient care.
These advancements underscore the importance of staying abreast of the latest statistical methodologies to drive progress in biostatistics.
Research Design Implications
In shaping the domain of biostatistics, the implications of research design wield profound influence, guiding the course of statistical analyses and insights.
The selection of an appropriate sample size is a critical aspect of research design, as it directly impacts the study's power and generalizability. Additionally, considering confounding variables at the design stage is essential to guarantee the validity of statistical inferences.
Data Analysis Evolution
Data analysis evolution heralds a transformative era in the domain of biostatistics, reshaping methodologies and augmenting the depth of statistical insights. In understanding this evolution, it's essential to contemplate the historical context and the evolutionary trends shaping the field.
Here are three key aspects to ponder:
- Technological Advancements: The evolution of data analysis tools and software has revolutionized how biostatisticians handle and interpret vast datasets.
- Interdisciplinary Collaboration: Increasingly, biostatistics is merging with other disciplines like computer science and data science, broadening its scope and impact.
- Adaptation to Big Data: The exponential growth of data requires biostatisticians to continually refine their methods to extract meaningful conclusions in the era of big data.
Frequently Asked Questions
How Does the Book Address the Issue of Missing Data in Biostatistics Analysis?
In biostatistics, handling missing data is vital. Imputation techniques like Multiple Imputation are used to address this issue. Sensitivity analysis helps assess the impact of missing data on results, ensuring robustness in statistical analyses.
Are There Any Discussions on the Ethical Considerations in Conducting Biostatistical Studies?
Ethical considerations in biostatistical studies are important. Ensuring data completeness upholds integrity. The book may explore these topics, offering valuable insights on maneuvering the complex intersection of statistics and ethics in research.
Does the Book Provide Guidance on How to Choose the Most Appropriate Statistical Test for a Given Research Question in Biostatistics?
In traversing the statistical landscape, one must consider the research design's intricacies, statistical assumptions, and hypothesis testing. Decision making on the most suitable statistical test is pivotal, ensuring the integrity of biostatistical analyses.
Are There Any Recommendations on How to Effectively Communicate Statistical Findings to Non-Statistical Audiences in the Field of Biostatistics?
Effective communication of statistical findings to non-statistical audiences in biostatistics requires clarity, simplicity, and relevance. Recommendations include using plain language, visual aids, and real-world examples to convey complex concepts in an understandable manner.
Does the Book Discuss the Potential Limitations and Biases That Can Arise in Biostatistics Studies and How to Mitigate Them?
In biostatistics, understanding limitations, biases, and ethical considerations is essential. Mitigation strategies like sensitivity analysis for missing data and randomization help address these issues. By acknowledging and managing these factors, researchers guarantee robust, reliable study outcomes.