The Analysis of Biological Data by Michael C. Whitlock and Dolph Schluter – Summary and Review
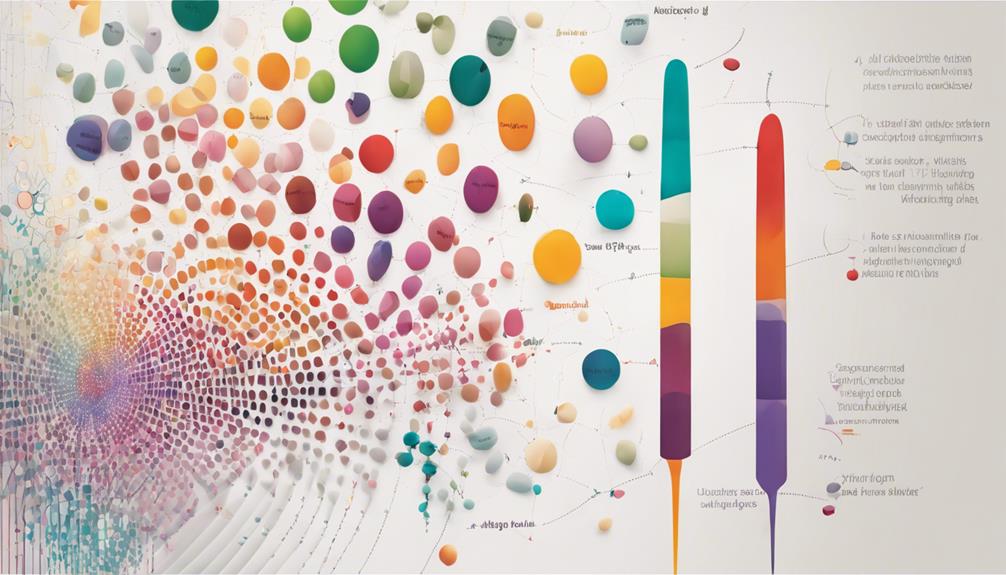
'The Analysis of Biological Data by Whitlock and Schluter' is a comprehensive guide to statistical methods and hypothesis testing in biology. It provides practical tools and professional insights for researchers. The book offers a profound understanding and interpretation of biological data beyond academia. It is relevant for both students and seasoned scholars seeking enlightenment in biological inquiry.
Key Takeaways
- Statistical methods in biology reveal patterns in data.
- Understanding statistical techniques aids in scientific discovery.
- Data visualization uncovers relationships in biological datasets.
- Robust data analysis ensures accurate research conclusions.
Overview of Statistical Methods
In the domain of scientific inquiry, statistical methods serve as the essential toolkit for analyzing and interpreting biological data. Data visualization methods play a critical role in exposing patterns and trends that might otherwise remain hidden within the vast complexity of biological datasets. Exploratory data analysis, a dynamic and iterative process, allows researchers to dive into their data, discovering insights and generating hypotheses that can guide further investigation.
Descriptive statistics, another cornerstone of statistical analysis, provide a concise summary of key features of the data, offering a snapshot of its central tendencies, dispersion, and shape. Through the application of data transformation techniques, researchers can manipulate the data to meet the assumptions of statistical tests, ensuring the validity and reliability of their findings.
Key Concepts Discussed
The crux of statistical techniques within the domain of biology unfolds a tapestry of understanding that interweaves theory with practical applications.
The intricate dance between statistical methods and biological data illuminates patterns and relationships that offer profound insights into the natural world.
Within this symbiotic relationship lies the potential to unravel mysteries, foster discovery, and cultivate a deeper appreciation for the interconnectedness of science and life.
Statistical Techniques Overview
Amidst the vast array of statistical techniques available for analysis in biology, a foundational understanding of key concepts is essential for researchers to effectively interpret and draw meaningful conclusions from their data.
Data visualization techniques play a pivotal role in elucidating patterns and relationships within complex datasets, aiding in the initial exploration and identification of trends.
Additionally, experimental design considerations are paramount in ensuring the validity and reliability of study outcomes, guiding researchers in the formulation of hypotheses and the execution of experiments.
Applications in Biology
Exploring the world of biological applications demands a keen understanding of how statistical techniques can be effectively employed to extract valuable insights from complex datasets. Data visualization techniques play a significant role in elucidating patterns and trends within biological data, aiding researchers in uncovering meaningful relationships.
Additionally, robust data analysis strategies are essential for drawing accurate conclusions and making informed decisions in various biological contexts. Experimental design serves as the foundation for generating reliable data, ensuring that research outcomes are both valid and reproducible.
Moreover, implementing sound data management practices is essential for organizing and maintaining the vast amounts of biological data generated in modern research. By integrating these key elements, researchers can navigate the intricate landscape of biological applications with precision and clarity.
Hypothesis Testing Techniques
In the domain of statistical analysis, mastery of hypothesis testing techniques is important for drawing meaningful conclusions from data. When conducting hypothesis tests, researchers often consider factors such as power analysis and effect size to ensure the validity and reliability of their findings. Power analysis helps determine the likelihood of detecting an effect if it truly exists, while effect size quantifies the magnitude of the difference observed. These components play a crucial role in the design and interpretation of experiments, allowing researchers to make informed decisions based on statistical evidence.
To further illustrate the importance of hypothesis testing techniques, the table below highlights key aspects related to power analysis and effect size:
Key Aspect | Description | Importance |
---|---|---|
Power Analysis | Evaluates the probability of detecting a true effect when it exists, helping researchers avoid false negative results. | Ensures study results are reliable. |
Effect Size | Measures the strength of the relationship between variables, providing insight into the practical significance of findings. | Helps interpret the magnitude of effects. |
Regression Analysis Applications
Within the domain of biological data analysis, the applications of regression hold immense significance. Understanding how regression aids in interpreting data and its important implications in experimental settings is vital.
These points will shed light on the pivotal role regression analysis plays in the field of biology.
Regression in Biology
With precision and elegance, regression analysis serves as a powerful tool in unraveling the intricate relationships between biological variables. When exploring linear relationships and model selection in biology, researchers often utilize regression to understand the influence of predictor variables and their biological significance. This statistical technique allows for the quantification of the relationship between variables, providing valuable insights into biological processes. By fitting a regression model to the data, scientists can make predictions and test hypotheses, aiding in the interpretation of complex biological phenomena. Below is a table highlighting the key aspects of regression analysis applications in biology:
Regression Analysis Applications |
---|
Explore linear relationships |
Aid in model selection |
Quantify predictor variables |
Uncover biological significance |
Enhance data interpretation |
Data Interpretation Techniques
Regression analysis applications extend beyond exploring linear relationships and model selection in biology to encompass sophisticated data interpretation techniques that illuminate the biological significance of predictor variables.
By utilizing data visualization tools, researchers can effectively communicate complex relationships between variables. This visualization aids in understanding patterns that might otherwise be obscured in raw data.
Additionally, sound experimental design is vital when applying regression analysis to guarantee the validity and reliability of the results. Properly designed experiments enhance the accuracy of the relationships identified by regression models.
Through these techniques, researchers can extract valuable insights from biological data, shedding light on the intricate relationships within biological systems.
Practical Experimental Applications
How can researchers effectively apply regression analysis techniques to practical experiments in biology to uncover meaningful relationships between variables? When it comes to experimental design and data analysis, the following key points can guide scientists in utilizing regression analysis effectively:
- Clear Hypotheses: Formulate precise hypotheses before conducting experiments to assure the regression analysis addresses specific research questions.
- Variable Selection: Carefully select variables based on biological relevance and avoid multicollinearity to obtain accurate and interpretable results.
- Model Validation: Validate regression models using techniques like cross-validation to assess their predictive power and generalizability.
- Interpretation: Interpret regression results cautiously, considering the biological context and potential confounding variables for robust conclusions.
Interpretation of Biological Data
Interpreting biological data reveals the intricate patterns and relationships hidden within the complex web of biological systems. Through data visualization approaches and data mining techniques, researchers can uncover valuable insights from vast datasets.
Model selection methods play a critical role in determining the most appropriate statistical approach to analyze the data, ensuring the reliability and accuracy of the conclusions drawn.
Experimental design strategies are essential for guiding the collection of data in a structured manner, ensuring that the results obtained are robust and meaningful.
Practical Tools for Researchers
Exploring practical tools empowers researchers to efficiently navigate the complexities of analyzing biological data and extracting meaningful insights from their research endeavors. When it comes to handling biological data, researchers benefit greatly from a set of tools that streamline their workflow and enhance the quality of their analyses.
- Data visualization strategies: Leveraging innovative visualization techniques allows researchers to present their findings in a clear and compelling manner, aiding in the communication of complex biological concepts.
- Data analysis techniques: Equipping researchers with a diverse toolkit of analytical methods enables them to uncover patterns, trends, and relationships within their datasets, leading to robust conclusions and scientific discoveries.
- Experimental design considerations: Carefully planning and executing experiments is important in ensuring the validity and reliability of research outcomes, ultimately enhancing the credibility of scientific findings.
- Data management practices: Implementing effective data management strategies facilitates the organization, storage, and retrieval of large volumes of biological data, promoting efficiency and reproducibility in research efforts.
Relevance for Students
For students, understanding the practical applications of biological data analysis is essential for fostering a strong foundation in scientific research. Student engagement in statistical analysis is paramount in today's educational landscape. 'The Analysis of Biological Data' by Whitlock and Schluter offers a detailed guide that can serve as a valuable educational resource for students delving into the domain of biological research.
By grasping statistical techniques and their application in analyzing biological data, students can enhance their critical thinking skills and develop a deeper understanding of complex biological processes. This book not only equips students with the necessary tools to conduct meaningful research but also instills confidence in their ability to interpret and draw conclusions from data.
Through hands-on practice and real-world examples, students can bridge the gap between theoretical knowledge and practical application, preparing them for success in various scientific endeavors. Embracing the principles outlined in this book can empower students to become proficient in statistical analysis, paving the way for a promising future in biological research.
Professional Insights and Recommendations
Drawing upon decades of experience in the field, seasoned professionals offer invaluable insights and strategic recommendations for excelling in the domain of biological data analysis.
- Continuous Learning: Embrace a mindset of lifelong learning to stay abreast of the ever-evolving tools and techniques in data analysis.
- Networking: Build a strong professional network within the scientific community to foster collaborations, share knowledge, and open up new career opportunities.
- Specialization: Consider specializing in a niche area of biological data analysis to become an expert in a particular field, increasing your value and demand in the job market.
- Communication Skills: Develop strong communication skills to effectively convey complex data analysis results to a broad audience, facilitating better understanding and decision-making.
Frequently Asked Questions
How Does the Book Address the Ethical Considerations and Challenges of Working With Biological Data?
When addressing ethical considerations and challenges of working with biological data, the book navigates complex terrain with clarity. It encourages a thoughtful approach, highlighting the importance of integrity in research and the need for responsible data management.
Are There Any Case Studies or Real-Life Examples Included in the Book to Demonstrate the Application of Statistical Methods in Biological Research?
The book presents case studies and real-life examples to vividly demonstrate the practical applications of statistical methods in biological research. These instances offer a clear understanding of how to apply these tools effectively in scientific investigations.
What Are Some Common Pitfalls or Mistakes That Researchers Often Make When Analyzing Biological Data, and How Does the Book Address Them?
Researchers commonly fall into pitfalls in statistical analysis and data interpretation, such as overlooking assumptions or misapplying tests. The book adeptly navigates these challenges by offering clear explanations, practical examples, and guidance on proper methodology to enhance research integrity.
How Does the Book Discuss the Importance of Collaboration and Interdisciplinary Approaches in Analyzing Biological Data?
In discussing the importance of collaboration and interdisciplinary approaches in analyzing biological data, the book emphasizes the power of diverse perspectives to tackle complex challenges. It highlights how collaborative research enhances data ethics and addresses various analytical hurdles.
Can You Provide Some Insights or Recommendations From the Authors on How Researchers Can Effectively Communicate and Present Their Findings Using Statistical Analysis in the Field of Biology?
Researchers should prioritize clear data visualization for effective statistical communication in biology. By focusing on interpretation and presentation skills, they can convey findings concisely and engage audiences. Such practices enhance collaboration and interdisciplinary understanding in the field.